Development of High-precision Thermosphere Models for Improving Precise Orbit Determination of Low-Earth-Orbiting Satellites (TIPOD)
Principal investigator: Jürgen Kusche
Project manager/Main research: Armin Corbin
Title of research project: Development of High-precision Thermosphere Models for Improving Precise Orbit Determination of Low-Earth-Orbiting Satellites (TIPOD)
Funding: DFG-SPP1788
Project partners: University of Bonn, DGFI-Technical University of Munich, Technical University of Munich, German Research Centre of Geosciences
Project endurance: Jan 2018 - Dec 2021
Project area: Geophysics and Geodesy (315)
Cluster: bonna
Software: gcc, open MPI, NetDCF, PDAF, TIE-GCM, ESMF, ParaView, Panoply
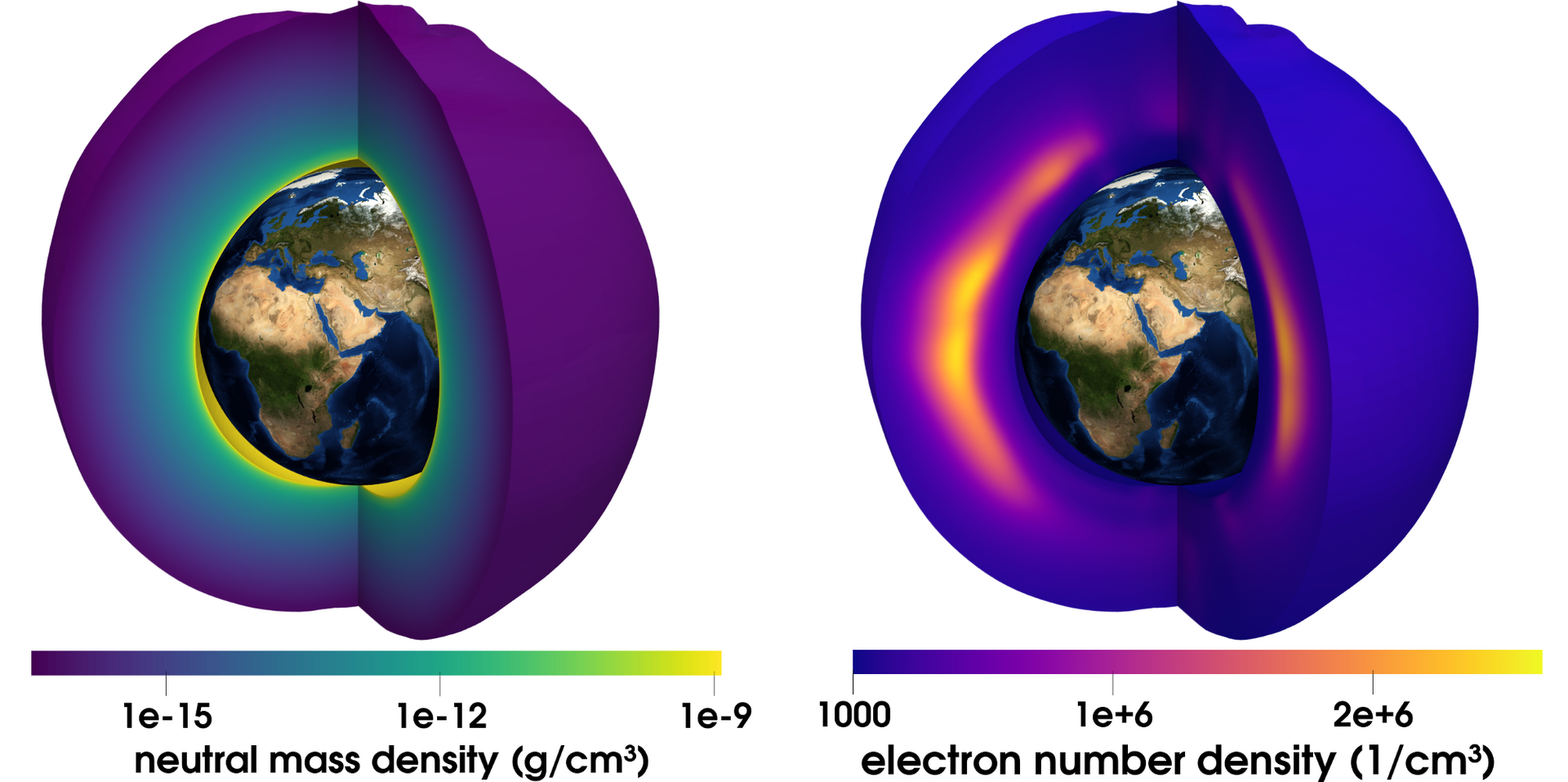
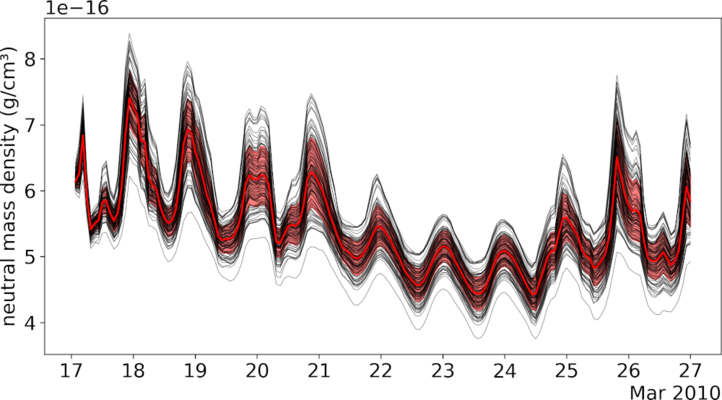
Introduction:
Anyone who has ever ridden a bicycle or a roller coaster, has experienced atmospheric drag deceleration: The particles in the air collide with our body and slow us down. One parameter affecting the magnitude of the atmospheric drag deceleration is the density of the atmosphere. Essentially, this means that more particles per volume lead to larger deceleration. Air density decreases approximately exponentially with height. For instance, the air density at 350 km altitude is twelve orders of magnitude lower than at ground level. That means the number of particles is a trillion times smaller. Nevertheless, there are enough particles at this altitude to decelerate satellites significantly. The atmospheric drag deceleration also depends on the collision velocity, the surface area, and the materials of the satellite. The success of some satellite missions depends on a precise determination of the satellite’s orbit. As an example consider a satellite measuring the sea level using radar. Every error in the vertical position of the satellite directly propagates into the measured sea level. Thus, one has to consider the atmospheric drag deceleration for the highest accuracy. Once a satellite has run out of fuel it cannot compensate the drag, loses speed and comes closer to Earth, accordingly. Eventually it disintegrates, pieces burn up or sometimes fall into the ocean. To predict the crash location and to assess the lifetime of satellites precise knowledge of the atmospheric density is required. The density of the atmosphere is subject to spatial and temporal variations. There are several empirical and physical models providing the density. However, there are significant differences between those models and also observations of the density. Our goal is to improve models by combining them with observations using data assimilation.
Methods:
Data assimilation is a method that combines the estimate of a model with observations, taking into account the uncertainties of both. The result is an estimate with higher accuracy. We use a so called Ensemble Kalman Filter. This means we run many different versions of a model of the upper atmosphere with slightly different settings. For example, the Sun in one of the ensemble members may emit more/less radiation, the lower boundary may be warmer/cooler, the winds may be faster/slower, or electric currents in the atmosphere may produce more/less heat. The spread of the ensemble members reflects the uncertainties of the model. We have coupled the Thermosphere Ionosphere Electrodynamics General Circulation Model (TIEGCM) developed at the NCAR High Altitude Observatory with the Parallel Data Assimilation Framework (PDAF) developed at the Alfred Wegener Institute. This enables us to perform data assimilation experiments.
Results:
In Figure 1 one can see the neutral density and electron density of the Thermosphere computed by the TIE-GCM. Figure 2 shows the ensemble spread of the neutral density.
Discussion:
Each TIE-GCM instance (each ensemble member) can be computed on up to 80 cores. Since all instances are computed in parallel it is easy to use several thousand cores to capacity. There is an infinite number of configurations for the data assimilation setup. The most important settings are the setup of the ensemble, the choice of the (Ensemble Kalman) Filter and the choice of observations.
Outlook:
Currently, we assimilate the output of empirical models that are fitted from observations, since they are available for any location and time. This will lead us later to the direct assimilation of observations which is more challenging since satellite along-track data is very sparse. By reason of limited computational resources we currently evaluate the TIE-GCM on a 5 grid. However, we plan to use a 2.5 grid in the future.
[1] Kristin Vielberg, Armin Corbin, Jürgen Kusche, Chao Xiong, and Claudia Stolle. under review. Ionosphere-thermosphere relation as seen in measured and modeled electron and neutral densities. Earth, Planets and Space.
[2] Kristin Vielberg, Christina Lück, Armin Corbin, Ehsan Forootan, Anno Löcher, and Jürgen Kusche. 2021. TND-IGG RL01: Thermospheric neutral density from accelerometer measurements of GRACE, CHAMP and Swarm. data set. (2021). doi: 10 . 1594 / PANGAEA . 931347. doi.org/10.1594/PANGAEA.931347.
[3] Zeitler, L., Corbin, A., Vielberg, K., Rudenko, S., Löcher, A., Bloßfeld, M., et al. (2021). Scale factors of the thermospheric density: A comparison of satellite laser ranging and accelerometer solutions. Journal of Geophysical Research: Space Physics, 126, e2021JA029708. https://doi.org/10.1029/2021JA029708
[4] Corbin, A., Kusche, J. Improving the estimation of thermospheric neutral density via two-step assimilation of in situ neutral density into a numerical model. Earth Planets Space 74, 183 (2022). https://doi.org/10.1186/s40623-022-01733-z